AI Enablement & Automation: Turning high-friction, manual workflows into smart, scalable systems that lift productivity without bloating teams
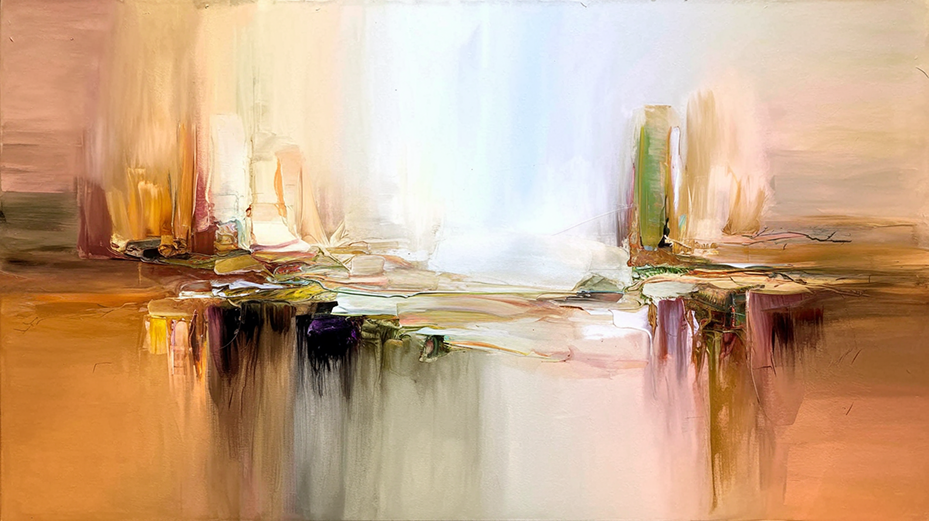
Modern organisations are drowning in copy-and-paste work. Knowledge workers still spend entire afternoons triaging email threads, re-keying data between SaaS tools, or searching SharePoint for the “right” version of a slide.
That friction is now a strategic risk: McKinsey’s latest State of AI survey finds that 78% of companies already deploy AI in at least one business function – up from 55% only a year ago (The State of AI: Global survey | McKinsey). The longer mid-market operators wait, the further the productivity gaps will yawn.
Mindlace focuses on helping companies in seven key moments. This post unpacks Moment 1 – AI Enablement & Automation: the point where firms decide to swap swivel-chair processes for intelligent workflows that free people to do work that moves the needle. See a summary of those seven moments here.
Why the automation moment matters now
- The work-hour opportunity – By 2030 up to 30% of hours now worked across the economy could be automated (Generative AI and the future of work in America | McKinsey), and 60% of occupations already have at least one-third of tasks that could be handed to software robots or agentic AI (Jobs of the future: Jobs lost, jobs gained - McKinsey & Company). That’s slack in the system waiting to be redeployed toward growth.
- CEO urgency – In IBM’s 2024 CEO benchmark, 67% of chief executives say the productivity gains from automation are so great they will “accept significant risk” to secure them (IBM Study: 6 hard truths CEOs must face).
- Real capacity unlocked – UiPath’s own automation centre saved its 4,000 staff 88,800 hours in one quarter – more than a decade of human effort every three months (How UiPath Robots save our employees 7400 hours every week).
These numbers expose a widening delta between companies that automate by design and those that keep patching processes with headcount.
Signals you’ve reached Moment 1
- “We need an AI plan—yesterday.” A 2024 McKinsey poll found only 11% of executives believe they have a fully implemented, responsible-AI capability, yet 92% expect to increase AI investment over the next three years—an anxiety gap between intent and roadmap. McKinsey & Company
- Pilot purgatory. IDC reports that 88% of AI proof-of-concepts never make it into production, leaving stranded cost and shattered morale. CIO
- Too many spreadsheets, not enough insights. Deloitte’s Q3 2024 enterprise study shows 68% of firms have moved fewer than a third of Gen-AI pilots into real workflows, largely because data still lives in Excel silos. Medium
- The four-person “AI team”. BCG notes that most mid-market operators advertise for a lone data-scientist pod (< 5 people) while expecting enterprise-level impact—a classic under-resourcing tell-tale. BCG Global
- RPA graveyards. Bots built last year break whenever the UI nudges, forcing IT to babysit scripts rather than retire toil—an unmistakable sign you need integrated, AI-first automation.
- Shadow SaaS stack. Ops staff pay for Zapier or Airtable out-of-pocket to keep processes moving. When workarounds proliferate, core systems are crying out for intelligent orchestration.
- Service-level slippage. Ticket queues lengthen while hiring is frozen; customer-support wait-times stretch beyond SLA targets. Automation is now the only realistic headcount lever.
- Audit and compliance drag. Manual reconciliations dominate month-end close; CFOs flag rising “spreadsheet risk” in board packs.
- C-suite spotlight. When CEOs start demo-ing ChatGPT in town-halls, expectation pressure on middle management spikes—forward-thinking teams secure budget before the hype turns hostile.
- Market peer moves. Competitors announce AI copilots or automated claims portals—your sales team begins fielding “do you have this too?” questions.
- Fractured data lineage. Analysts spend more time cleaning than analysing; you launch a “single source of truth” project every spring and still live in CSV purgatory.
- Security or privacy scares. Employees share sensitive data with public LLMs because official channels feel slower; risk teams escalate, signalling it’s time for governed, in-house automation agents.
If any of the above rings true, you are paying an “automation tax” every day.
How winning firms automate without the chaos
- Start with a workflow census – Map journey time, not just cycle time. Hidden hand-offs typically multiply effort by 4-6×.
- Pick “one-week payback” use cases – Target tasks where the robot time saved exceeds dev time inside seven days. Claims triage, invoice matching, Level-1 support macros.
- Combine narrow AI & RPA – LLMs classify, summarise or draft; bots push the result through legacy screens or APIs. The mix slashes failure rates versus LLM-only chatbots.
- Instrument everything – Treat every automated step as a data exhaust. That telemetry feeds next-wave optimisation and decision acceleration.
Mindlace fans this automation flywheel with Pathforger – the same framework we used to cut charter-management admin 80% for a luxury-yacht SaaS scale-up and prototype an AI-assisted re-pricing flow agent in six weeks for a UK-based Risk Agency.
Common pitfalls (and how to dodge them)
- Bot-sprawl without governance. RPA scripts keel over as soon as a UI shifts one pixel. Fix: manage automations as “infrastructure-as-code”, apply version control, and promote each bot through dev / test / prod just like micro-services.
- LLM vanity projects. A shiny GPT wrapper that demos well but actually adds steps for users. Fix: benchmark end-to-end lead time, and kill any build that doesn’t shorten it.
- Change fatigue. Automations that land without enablement stall out. Fix: pair every bot launch with a 30-minute team clinic and a Slack/Teams channel for real-time tweaks.
- Automating a bad process. If the workflow is broken, a robot just helps you make mistakes faster. Fix: run a rapid value-stream mapping first—eliminate steps, then automate what’s left.
- Security & compliance blind spots. LLMs can leak personal or proprietary data if prompts aren’t sanitised. Fix: enforce data-classification gates, redact sensitive fields at ingestion, and keep a human-in-the-loop for any regulated output.
- Model drift and silent failures. An AI classifier that worked on last quarter’s data degrades quietly, eroding user trust. Fix: establish MLOps monitoring—accuracy dashboards, auto-retraining triggers and rollback scripts baked into CI/CD.
- Ownership vacuum. Bots become “orphan tech” when the champion moves teams, and no one patches them. Fix: assign a business owner plus a technical steward for every automation, with KPIs tied to uptime and value delivered.
- Fuzzy success metrics. Without a baseline, claimed productivity wins feel like smoke. Fix: quantify current effort (hours, error rate, cycle time) before you start; track those same metrics post-go-live and publicise the deltas every sprint.
Addressing these traps up-front is how Mindlace ships AI-powered automations that stay live—delivering compounding productivity instead of one-off fanfare.
Ready to release your people from copy-and-paste purgatory? Book a discovery call with Mindlace. In 90 days you’ll have live AI agents, measurable hours back - and a culture that never settles for swivel-chair work again.
The Mindlace edge
- Small squads, big impact – 3-8 experts integrate with your tech, ops or other relevant teams and ship the first working agent in < 30 days.
- Impact Pledge – Every fortnightly sprint must show a quantifiable gain - or we waive the sprint fee.
- Built-in upskilling – We leave behind scripts, playbooks and on-call coaching so your people own the automations long after we exit.